报告题目:Monotonicity, A Versatile Property in Data Science
报告时间:2018年11月22日,星期四,下午3:00-4:30
报告地点:北五楼427
报告人:Bernard De Baets,senior full professor,Department of Data Analysis and Mathematical Modelling,Ghent University
报告摘要:
In many modelling problems, there exists a monotone relationship between one or more of the input variables and the output variable, although this may not always be fully the case in the observed input-output data due to data imperfections. Monotonicity is also a common property of evaluation and selection procedures. In contrast to a local property such as continuity, monotonicity is of a global nature and any violation of it is therefore simply unacceptable. We explore several problem settings where monotonicity matters, including fuzzy modelling, machine learning and decision making. Central to the above three settings is the cumulative approach, which matches nicely with the monotonicity requirement.
By far the most popular fuzzy modelling paradigm, despite its weak theoretical foundations, is the rule-based approach of Mamdani and Assilian. In numerous applied papers, authors innocently assume that given a fuzzy rule base that appears monotone at the linguistic level, this will be the case for the generated input-output mapping as well. Unfortunately, this assumption is false, and we will show how to counter it. Moreover, we will show that an implication-based interpretation, accompanied with a cumulative approach based on at-least and/or at-most quantifiers, might be a much more reasonable alternative.
Next, we deal with a particular type of classification problem, in which there exists a linear ordering on the label set (as in ordinal regression) as well as on the domain of each of the features. Moreover, there exists a monotone relationship between the features and the class labels. Such problems of monotone classification typically arise in a multi-criteria evaluation setting. When learning such a model from a data set, we are confronted with data impurity in the form of reversed preference. We present the Ordinal Stochastic Dominance Learner framework, which permits to build various instance-based algorithms able to process such data.
Finally, we explore a pairwise preference setting where each stakeholder expresses his/her preferences in the shape of a reciprocal relation that is monotone w.r.t. a linear order on the set of alternatives. The goal is to come up with an overall monotone reciprocal relation reflecting `best' the opinions. We formulate the problem as an optimization problem, where the aggregated linear order is that for which the implied stochastic monotonicity conditions are closest to being satisfied by the distribution of the input monotone reciprocal relations. Interesting links with social choice will be pointed out.
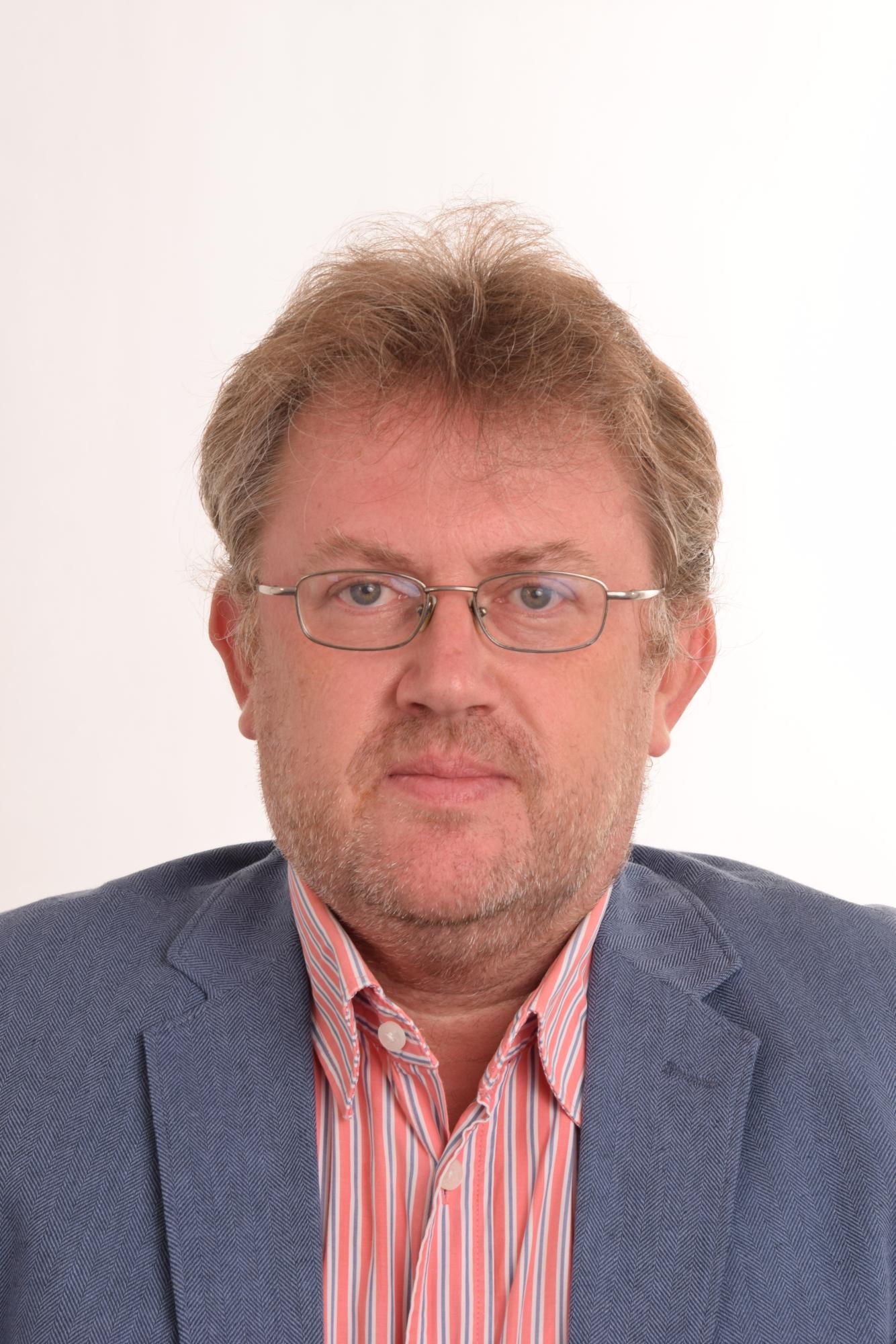
报告人简介:
Bernard De Baets is a senior full professor in applied mathematics at the Faculty of Bioscience Engineering (Shanghai rank 37 in Life and Agriculture Sciences) of Ghent University, the top-ranked Belgian university (Shanghai rank 61). He is leading the research unit KERMIT and acts as head of the Department of Data Analysis and Mathematical Modelling. Furthermore, he is an affiliated professor at the Anton de Kom Universiteit (Suriname), an Honorary Professor of Budapest Tech (Hungary), a Doctor Honoris Causa of the University of Turku (Finland) and a Profesor Invitado of the Universidad Central “Marta Abreu” de las Villas (Cuba).
As a trained mathematician, computer scientist and knowledge engineer, Bernard has developed a passion for multi- and interdisciplinary research. He is not only deeply involved in fundamental research in three interlaced research threads, namely knowledge-based, predictive and spatio-temporal modelling, but he also aims at innovative applications in the applied biological sciences. At present, over 30 researchers are involved in the activities of KERMIT. Over the past 20 years, 67 PhD students have graduated under his (co-)supervision.
Bernard is a prolific writer, with a bibliography comprising close to 500 peer-reviewed journal papers, 60 book chapters and 300 contributions to conference proceedings, accumulating over 18000 Google Scholar citations (h-index 67). Several of his works have been bestowed upon with a best paper award. Moreover, he is a much-invited speaker, having delivered over 250 lectures world-wide. In 2011, he was elected Fellow of IFSA (International Fuzzy Systems Association) and in 2012, he was a nominee for the Ghent University Prometheus Award for Research.
Bernard actively serves the research community, in particular as co-editor-in-chief of Fuzzy Sets and Systems and as member of the editorial board of several other journals, including the Internat. J. of Approximate Reasoning, Engineering Applications of Artificial Intelligence, and the Iranian J. of Fuzzy Systems. He is a member of the Administrative Board of the Belgian OR Society.